HuatuoGPT-o1: A Medical Reasoning LLM From China With OpenAI o1 Like Capabilities Is Here
A deep dive into the techniques that let HuatuoGPT-o1 outperform all other open-source LLMs on multiple medical benchmarks.
Reasoning with LLMs is currently a hot topic in AI.
OpenAI releasing their o
-series LLMs that are built for complex reasoning, with o-3 scoring 88% on a challenging benchmark created to test for AGI (called ARC-AGI), has made everyone curious about the secret behind these models.
While researchers are trying to decode the techniques that make o1 so powerful in open-source, their work is primarily based on mathematical reasoning tasks.
This is true for DeepSeek-R1 as well.
But the wait is over!
In the largely unexplored medical domain, where reasoning and reliability are absolutely important, a group of researchers has just released an LLM with o-1-like reasoning capabilities.
The training of this model, HuatuoGPT-o1, involves fine-tuning with advanced Chain of Thought (CoT) reasoning and further Reinforcement learning (RL) to enhance its abilities.
These make the LLM so good that it strikingly outperforms all other open-source general and medical-specific LLMs on multiple medical benchmarks.
Here’s a story in which we deep-dive and discuss step-by-step how HuatuoGPT-o1 was trained and what makes it perform so well.
Let’s begin!
Step 1: Preparing The Training Data
Most medical LLMs are trained on close-ended medical multiple-choice questions (MCQs).
These questions have limited options to choose an answer from and can be easily guessed by an LLM without requiring proper reasoning.
This needed to be fixed if we wanted a better-performing LLM.
Therefore, instead of training on close-ended MCQs, HuatuoGPT-o1 is trained on verifiable medical problems.
Let’s learn how this is done.
To create the training dataset, 192,000 MCQs are first selected from the MedQA-USMLE and MedMCQA datasets.
If any question from these can be correctly solved with three small LLMs (Gemma 29B, LLaMA-3.1–8B, Qwen2.5–7B), it is removed from the training dataset.
This is because such a question is considered not challenging enough to involve complex reasoning.
Alongside, questions asking for ‘incorrect options’ or with multiple correct or ambiguous answers are picked using GPT-4o and removed.
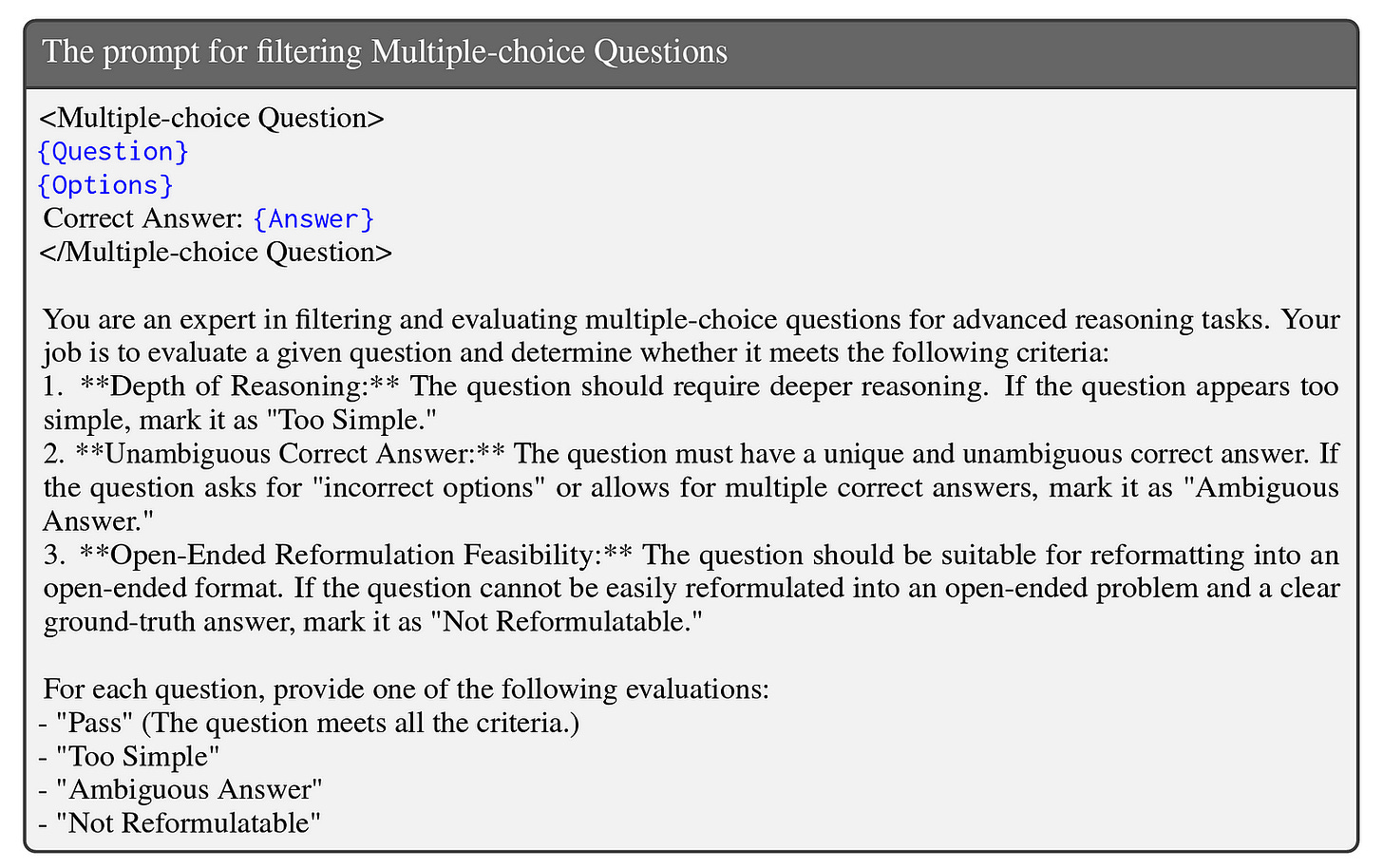
GPT-4o is again used to reformat each close-ended question into an open-ended one using the following prompt.
This results in the final training dataset consisting of 40,000 medical verifiable problems and their ground truth answers.
Each verifiable problem and its ground truth answer is denoted using x
and y*
, respectively.
Keep reading with a 7-day free trial
Subscribe to Into AI to keep reading this post and get 7 days of free access to the full post archives.