Revisiting The Basics: Rotary Position Embeddings (RoPE)
A lesson on Positional Embeddings from the ground up.
Transformers process tokens in parallel rather than sequentially.
This is what gives them the computational advantage over RNNs.
However, this also makes Transformers position-agnostic, meaning they do not have a sense of the order of the tokens they process.
Consider these two sentences:
“The cat sits on the mat.”
“The mat sites on the cat.”
To a Transfromer, both of them are the same.
This isn’t good for language processing.
Therefore, positional information in the form of positional embeddings (vectors) is added with token embeddings before Transformers process them.
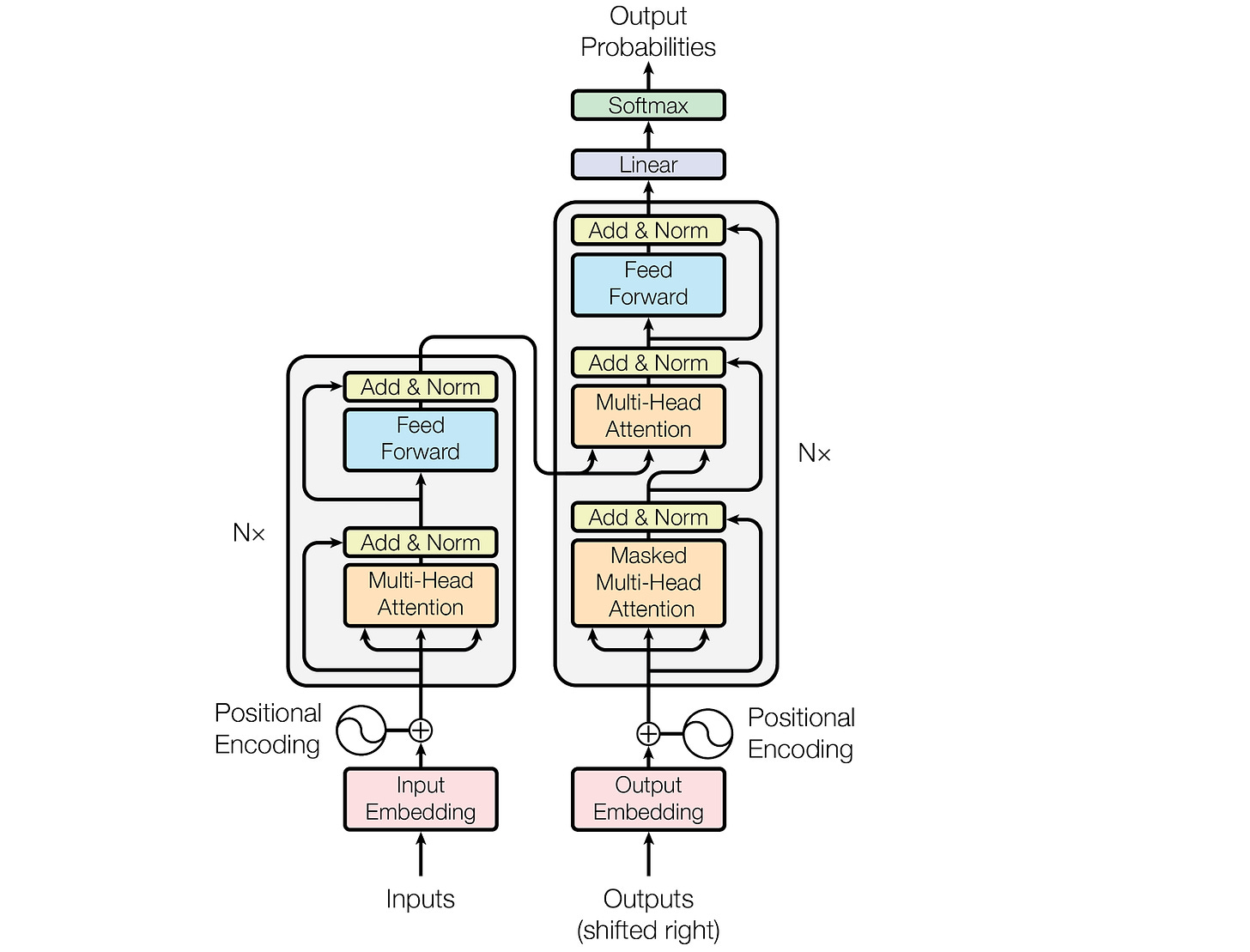
Keep reading with a 7-day free trial
Subscribe to Into AI to keep reading this post and get 7 days of free access to the full post archives.